The Hidden Power of Data Visualization
In today’s data-saturated business environment, the ability to extract meaningful insights from complex datasets isn’t just advantageous—it’s essential for survival. White label embedded analytics solutions have emerged as game-changers for SaaS providers looking to enhance their offerings without developing analytics capabilities from scratch. This approach allows companies to integrate sophisticated data visualization tools and dashboards directly into their existing platforms, all while maintaining their brand identity. According to research from Gartner, organizations that offer embedded analytics to their customers see up to 30% higher customer retention rates compared to those without such features. Just as AI voice assistants have transformed customer service interactions, embedded analytics is reshaping how businesses present data to their clients.
What Exactly Is White Label Embedded Analytics?
White label embedded analytics refers to the integration of third-party analytics tools into your software platform under your own branding. Think of it as a sophisticated dashboard system that lives inside your application, providing users with interactive charts, graphs, and data exploration capabilities without ever having to leave your ecosystem. Unlike traditional business intelligence tools that require users to switch between applications, embedded analytics creates a seamless experience where data insights become an organic part of your software’s workflow. This integration approach mirrors the convenience that AI call centers provide by keeping customer interactions within a unified system, eliminating friction and enhancing the user experience substantially.
The Business Case for Branded Analytics
Adding analytics capabilities to your SaaS platform isn’t just about following trends—it directly impacts your bottom line. When businesses incorporate white label analytics solutions, they typically see a 20-40% increase in user engagement and substantial boosts in subscription renewal rates. The numbers tell a compelling story: according to a study by Aberdeen Group, companies offering embedded analytics command premium pricing, with an average of 24% higher per-user revenue than competitors without such features. This value proposition resembles how AI phone agents allow businesses to offer premium customer service experiences that justify higher service fees. By transforming raw data into actionable insights within your branded environment, you’re not just selling software—you’re selling business intelligence as a value-added service.
Key Features That Make White Label Analytics Shine
The most effective white label analytics solutions come equipped with features designed to maximize both usability and insight generation. Look for platforms offering customizable dashboards that adjust to different user roles, interactive data visualization tools that allow for drill-down exploration, and real-time analytics capabilities that keep information fresh and relevant. Advanced offerings include predictive analytics functions, automated report generation, and multi-device responsiveness for mobile access. Just as conversational AI systems adapt to different user needs, premier embedded analytics platforms adjust their complexity based on user sophistication, making data accessible to both novice users and data scientists within the same system.
Implementation Strategies: The Integration Roadmap
Successfully implementing white label analytics requires thoughtful planning and execution. Begin with a thorough assessment of your users’ analytics needs through customer interviews and usage pattern analysis. Next, select an analytics provider whose technical architecture aligns with your existing platform—whether that’s JavaScript-based, REST API integration, or iframe embedding. The implementation process typically involves four phases: technical integration, branding customization, user permission configuration, and performance optimization. This methodical approach mirrors the structured implementation process used for AI appointment schedulers, where careful planning ensures seamless adoption. Companies that rush this process often face user resistance and technical debt, so allocate sufficient resources for testing and refinement.
Customization: Making Analytics Truly Your Own
The "white label" aspect of embedded analytics is what transforms a third-party solution into a seamless extension of your brand. Leading providers offer extensive customization options, allowing you to align color schemes, fonts, and UI elements with your established brand guidelines. Beyond visual elements, you can customize terminology, report templates, and even the types of visualizations available to match your industry’s specific needs. Some platforms allow for custom coding extensions that enable unique analytical functions specific to your business domain. This depth of personalization is similar to how white label AI receptionists can be tailored to represent specific business personalities and communication styles, creating a cohesive brand experience across all touchpoints.
Security Considerations in Embedded Analytics
Data security isn’t optional when implementing embedded analytics—it’s fundamental. Robust white label solutions offer multi-layered security frameworks, including row-level data access controls that ensure users see only the data they’re authorized to access. Look for platforms with SOC 2 compliance, GDPR readiness, and encryption for both data at rest and in transit. Additionally, consider solutions that offer single sign-on (SSO) integration to streamline authentication while maintaining security protocols. The security challenges here parallel those faced when implementing AI calling solutions, where protecting sensitive conversation data requires rigorous safeguards. Regular security audits should be conducted to ensure that your embedded analytics maintain compliance with evolving data protection regulations.
User Adoption: The Make-or-Break Factor
Even the most sophisticated analytics solution fails if users don’t embrace it. Successful implementation requires a thoughtful adoption strategy that includes in-app tutorials, contextual help resources, and guided walkthroughs of key features. Progressive disclosure techniques—showing basic functions first before introducing advanced capabilities—can prevent overwhelming new users. Consider implementing a "champion user" program where early adopters help train others within their organizations. This approach to user adoption shares principles with AI sales representative onboarding, where gradual exposure to capabilities leads to higher utilization rates. Analytics providers that offer customer success services can significantly improve adoption rates through customized training programs and adoption benchmarking.
The ROI Equation: Measuring Analytics Success
Quantifying the return on investment from embedded analytics requires tracking both direct and indirect metrics. Direct measurements include subscription upgrades attributed to analytics features, reduced churn rates, and increased average revenue per user (ARPU). Indirect benefits manifest as increased user engagement (measured through session duration and frequency), higher NPS scores, and competitive win rates in sales situations. A comprehensive ROI analysis should also factor in operational efficiencies gained through automated reporting and reduced support tickets related to data questions. Similar to how AI sales calls can be evaluated through conversion metrics, embedded analytics should be assessed based on how effectively they drive desired business outcomes rather than just technical implementation success.
Industry-Specific Applications
Different industries leverage embedded analytics in unique ways to address sector-specific challenges. Healthcare organizations use embedded analytics to track patient outcomes and resource utilization, while maintaining strict HIPAA compliance. E-commerce platforms implement purchase pattern analytics that drive personalized recommendation engines. Financial service providers deploy risk assessment dashboards that combine multiple data sources to guide investment decisions. Manufacturing companies utilize real-time production analytics to minimize downtime and optimize supply chains. Just as AI calling bots for health clinics are specialized for medical scheduling nuances, embedded analytics solutions should be tailored to address the specific metrics and workflows that drive value in your particular industry.
The Technical Architecture Behind the Scenes
Understanding the technical underpinnings of embedded analytics helps in selecting the right solution for your needs. Most modern platforms operate on a microservices architecture that allows for modular implementation and scaling. Data processing typically happens through one of three models: client-side processing (fastest but limited to smaller datasets), server-side processing (more powerful but potentially slower), or hybrid approaches that balance performance with capabilities. Consider whether the solution uses its own data storage or connects to your existing data warehouses through ETL processes or direct connections. This technical consideration resembles the architecture decisions made when implementing SIP trunking solutions, where the underlying infrastructure significantly impacts performance, reliability, and scalability of the entire system.
Mobile Analytics: Insights on the Go
As business increasingly happens outside traditional office environments, mobile-optimized analytics have become essential. Leading white label solutions offer responsive design that automatically adjusts visualizations and controls for smaller screens without sacrificing functionality. Advanced mobile features include touch-optimized interactions, offline capabilities that cache recent data, and push notifications for critical metric changes. Some platforms even offer native mobile SDKs that allow for deeper integration with device capabilities like location awareness. This mobile-first approach parallels how AI voice agents are designed to function across multiple communication channels, ensuring consistent experiences regardless of how users access the system.
Predictive Analytics: From Insight to Foresight
The most sophisticated embedded analytics platforms are moving beyond descriptive analysis (what happened) to predictive capabilities (what will happen). These advanced features leverage machine learning algorithms to identify patterns in historical data and project future trends. For example, customer churn prediction models can identify at-risk accounts before they cancel, while sales forecasting tools can adjust projections based on multiple variables. Implementing predictive features requires careful attention to model accuracy and transparent communication about confidence levels. The underlying machine learning capabilities share technological DNA with Twilio’s AI assistants and similar conversational intelligence platforms, combining historical pattern recognition with probabilistic future projections.
Competitive Landscape: Choosing the Right Provider
The embedded analytics marketplace features established players like Tableau, Power BI, and Looker alongside specialized embedded analytics providers such as Sisense, Logi Analytics, and ThoughtSpot. When evaluating options, consider factors beyond feature lists: assess the vendor’s financial stability, development roadmap, and customer support reputation. Integration capabilities and documentation quality often make or break implementation timelines. Just as businesses carefully evaluate Vapi AI white label alternatives for voice AI implementations, conduct thorough due diligence on analytics providers, including reference checks with customers in your industry. Remember that switching costs can be high once you’ve implemented a solution, so prioritize long-term partnership potential over short-term cost savings.
Pricing Models and TCO Considerations
White label analytics providers offer various pricing structures that significantly impact total cost of ownership (TCO). Common models include per-user licensing, data volume tiers, feature-based pricing, or combinations thereof. When calculating TCO, factor in implementation costs, ongoing maintenance requirements, and internal resources needed for administration. Some providers offer consumption-based pricing that scales with actual usage, which can be advantageous for applications with unpredictable growth patterns. This pricing complexity mirrors the considerations when selecting SIP trunking providers, where apparent savings can be undermined by hidden costs and scaling inefficiencies. Request detailed cost projections for multiple growth scenarios to avoid budget surprises as your platform gains traction.
Integration with Other Business Systems
The true power of embedded analytics often emerges when connected to other business systems. Leading solutions offer pre-built connectors to popular CRM platforms, marketing automation tools, ERP systems, and custom databases. These integrations enable cross-functional analytics that provide holistic business views rather than data silos. For example, connecting sales analytics with marketing campaign data reveals true customer acquisition costs and ROI by channel. Similarly, operations data linked with financial metrics can uncover inefficiencies that impact margins. This integration philosophy shares common ground with how AI call centers connect with CRM systems to provide agents with contextual information during customer interactions.
Future Trends: Where Embedded Analytics Is Heading
The embedded analytics landscape continues to advance rapidly, with several key trends shaping future development. Augmented analytics—using AI to automatically discover insights and generate explanations—is reducing the expertise required to derive value from data. Natural language querying allows users to ask questions in plain English rather than building complex queries. Meanwhile, collaborative analytics features enable teams to share insights and annotations within the analytics interface itself. Edge computing capabilities are enabling real-time analytics even with intermittent connectivity. These innovations parallel developments in text-to-speech technology and other AI fields, where natural interfaces and automated intelligence are making sophisticated capabilities accessible to non-technical users.
Case Study: E-commerce Platform Success Story
A mid-sized e-commerce platform specializing in handcrafted goods implemented white label analytics to give their merchant sellers deeper insights into product performance. Before implementation, merchants relied on basic sales reports and had limited visibility into customer behavior. After integrating embedded analytics, sellers gained access to dashboards showing conversion funnels, customer demographics, and inventory optimization recommendations. Within six months, merchants using the analytics features saw 27% higher sales growth compared to non-users. The platform itself experienced a 35% reduction in merchant churn and was able to increase subscription prices by 15% for plans including advanced analytics. This success story demonstrates how embedded analytics created tangible value similar to how AI appointment booking bots transform scheduling efficiency for service businesses.
Common Implementation Pitfalls to Avoid
Despite the compelling benefits, embedded analytics projects can falter without proper planning. Common mistakes include underestimating data preparation requirements, neglecting user experience design, and failing to align analytics capabilities with actual business problems. Technical issues often arise from insufficient testing with realistic data volumes and inadequate attention to performance optimization. On the organizational side, lack of stakeholder alignment and insufficient training resources can hamper adoption. These implementation challenges echo the pitfalls encountered when deploying conversational AI for medical offices, where technical capability must be carefully balanced with practical usability within established workflows. Successful implementations typically involve cross-functional teams with representatives from product, engineering, data, and customer-facing departments.
Compliance and Governance Frameworks
As data regulations grow increasingly stringent, embedded analytics solutions must incorporate robust governance controls. Effective implementations include data lineage tracking that documents the origin and transformation of all metrics, centralized metadata management to ensure consistent definitions across reports, and audit logs that record who accessed what information. For industries with specific regulatory requirements like healthcare (HIPAA), finance (GLBA, PCI DSS), or European operations (GDPR), specialized compliance features may be necessary. This governance infrastructure resembles the compliance considerations for AI cold callers, where recording disclosures and data handling protocols must satisfy complex regulatory landscapes. Well-designed governance frameworks protect both you and your customers while enabling rather than inhibiting valuable data utilization.
Elevate Your SaaS Platform with Embedded Analytics
The integration of white label embedded analytics represents a strategic opportunity to transform your SaaS offering from a functional tool into an indispensable business intelligence platform. By providing users with actionable insights directly within your application, you create a compelling value proposition that justifies premium pricing and fosters deeper customer relationships. The implementation journey requires careful planning, thorough vendor evaluation, and ongoing optimization—but the competitive advantages are substantial and enduring. As data-driven decision making becomes the standard across industries, embedded analytics isn’t just a feature enhancement—it’s increasingly an expectation.
If you’re looking to enhance your business communications with the same level of sophistication, consider exploring Callin.io. This platform allows you to implement AI-powered phone agents to handle inbound and outbound calls autonomously. With Callin.io’s innovative AI phone agent, you can automate appointment setting, answer frequently asked questions, and even close sales through natural conversations with customers.
The free account on Callin.io offers an intuitive interface to set up your AI agent, with included test calls and access to the task dashboard for monitoring interactions. For those seeking advanced capabilities, such as Google Calendar integrations and built-in CRM functionality, subscription plans start at just $30 per month. Discover more about enhancing your business intelligence and communication capabilities at Callin.io.
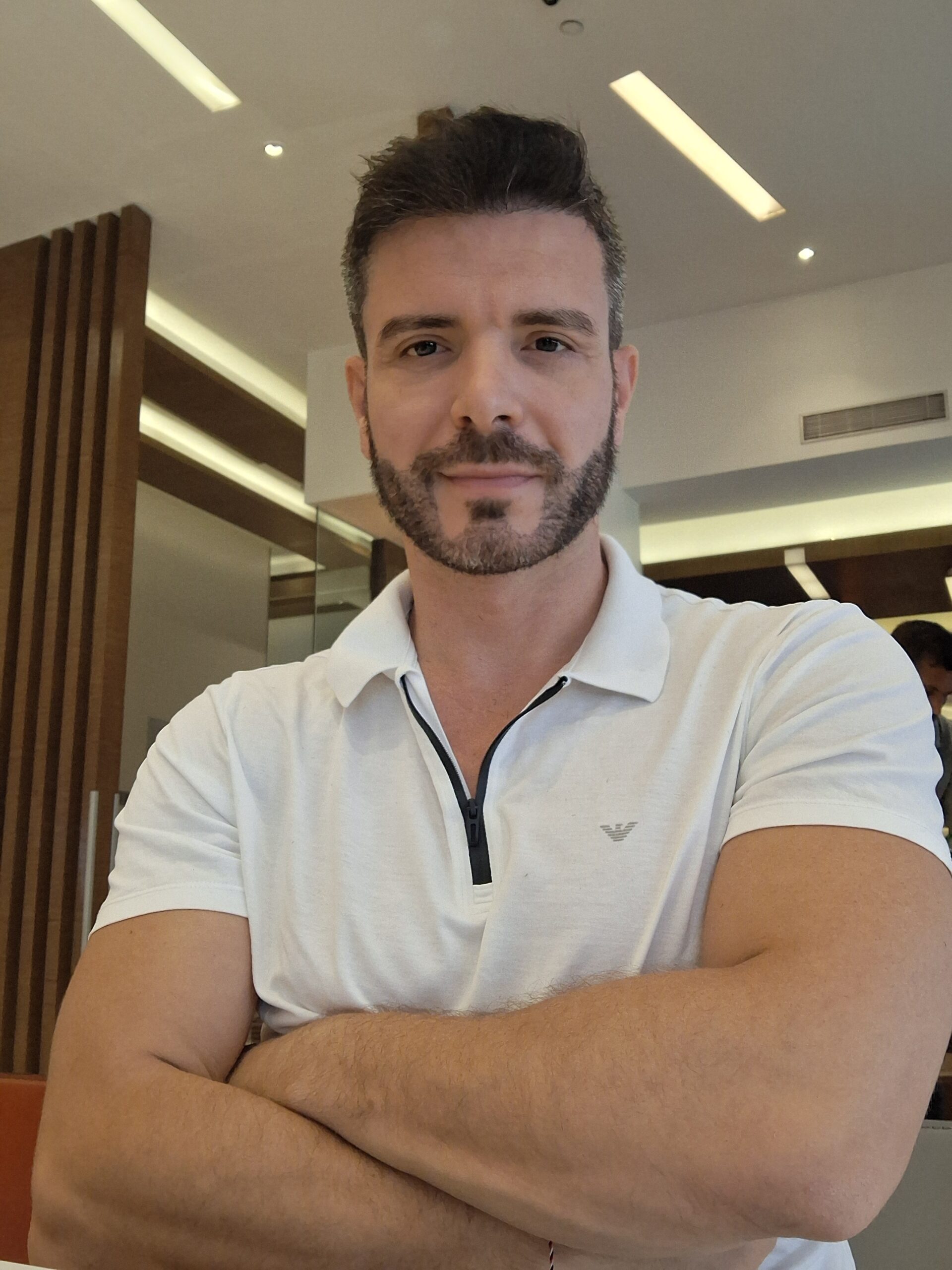
Helping businesses grow faster with AI. 🚀 At Callin.io, we make it easy for companies close more deals, engage customers more effectively, and scale their growth with smart AI voice assistants. Ready to transform your business with AI? 📅 Let’s talk!
Vincenzo Piccolo
Chief Executive Officer and Co Founder